How to Get Great Responses from RAG Tools: Content Curation
The success of a RAG system is deeply intertwined with the quality and curation of the data it's fed. By embracing the ethos of meticulous content curation, businesses can unlock the full potential of retrieval augmented generation tools
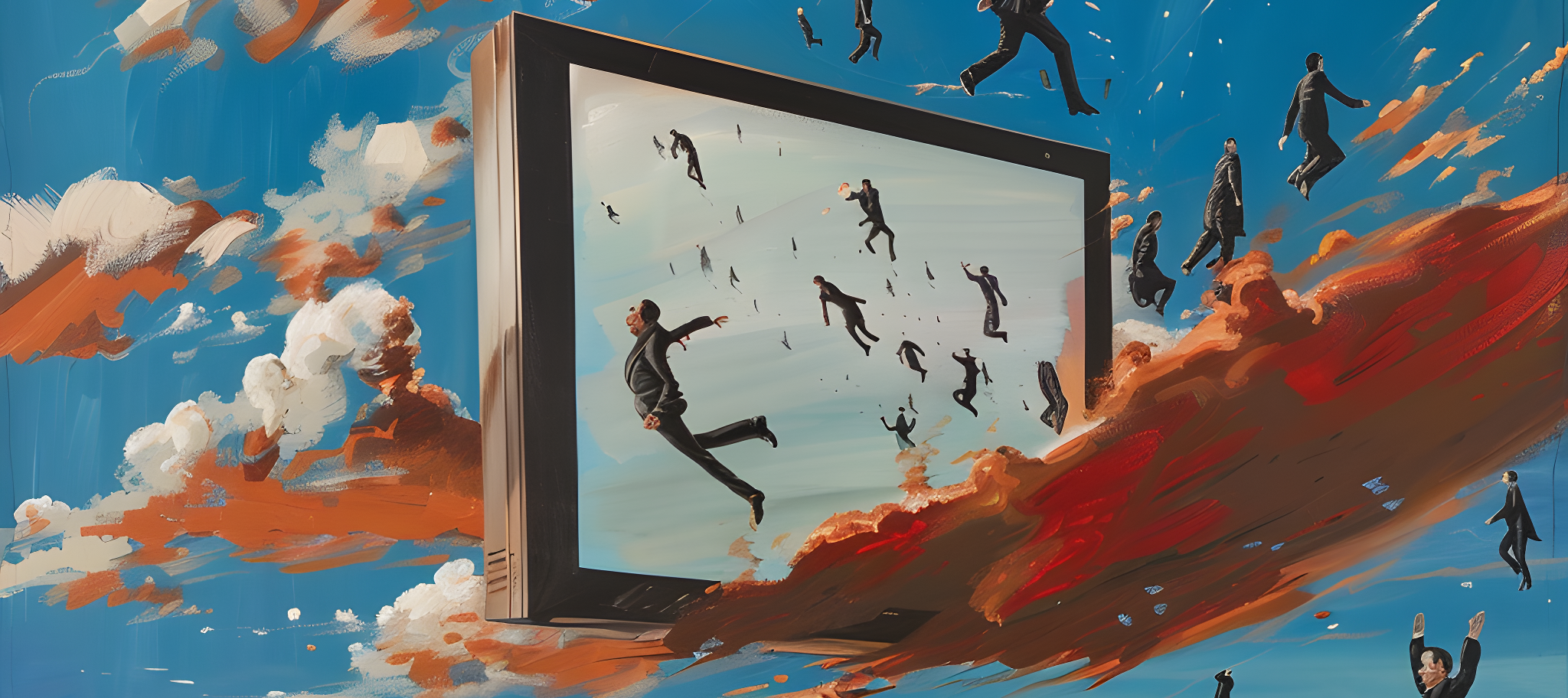
In software today, Large Language Models (LLMs) are everywhere. For now, they're not replacing humans, not least because the old adage of 'garbage in, garbage out' still holds true for LLM-powered products.
There's a certain kind of LLM-powered product that is heavily reliant upon careful knowledge curation. In RAG (retrieval augmented generation), the user uploads their own content to the system, and the system then leverages this content in order to answer user queries.
If you've used any kind of RAG tool, like MS Copilot, OpenAI's GPTs, MyAskAI, etc. then you'll have experienced how flimsy and unreliable responses can be. The most common reason for this is a lack of knowledge curation. In other words, uploading everything you've got and hoping for the best.
If you're looking for accurate, reliable, consistent responses, this is bad news.
Treat AI like a junior team member.
If you hired a new junior colleague tomorrow, how would you train them?
Certainly, you wouldn't throw hundreds of poorly organised documents their way and ask them to figure it out. You wouldn't then send them into the trenches to respond to high pressure requests from an eager, pushy sales team.
Equally, you shouldn't do this with a new RAG system.
Clean up your sources.
Poorly formatted, awkwardly structured sources can confuse LLMs. A simple rule of thumb is if it will confuse a human, it will confuse an LLM. The usual rules apply: clear paragraphs, simple formatting, spellcheck, headings & subheadings, etc.
Reduce noise.
Noise is the biggest threat to high quality responses from a RAG system. Anything you upload could be regurgitated by the system. And the more content you upload to a RAG system, the harder it is for the system to find the most important content. Eventually, finding the right answer becomes like finding a needle in a haystack.
One of the most impactful steps you can take is to be mindful of exactly what you are uploading to the system. Ask yourself, what kind of queries will this source serve?
Another risk of uploading a lot of content is that some content might conflict with other content. For example, if you upload a playbook describing how to negotiate a contract with a counterparty AND you upload your terms of service... less intelligent RAG systems will easily confuse what is allowed from a contractual perspective with what is allowed from a negotiation perspective.
Before deploying your RAG system to end users, consider if there are sources that might provide conflicting results. And if you need your system to handle these conflicts, consider a specialised tool (like Legal OS, DeepJudge, etc.).
Don't give up on RAG
RAG is mighty. Multiple Fortune 500 companies have already deployed RAG solutions at scale. They empower users to combine the impressive capabilities of foundation models (like ChatGPT) with grounding in their own content. For many use cases, this grounding is essential.
Of course, data curation isn't necessary with when directly querying foundation models such as ChatGPT. However, these tools give admins no control over the output and do not empower users to based responses on their own knowledge base.
For most meaningful business use cases, grounding in the company's knowledge base is table stakes. Even tasks like document review and email generation, which ChatGPT appears to handle reasonably well, the outputs are much more accurate and controllable when given solid grounding in company specific data. Otherwise users are reliant upon the original training data of the foundation model to inform responses... and in most cases, we're not even sure what that data is.
As a user of RAG, you control the data that goes in.
Instead of relying on AI providers (such as Open AI and Anthropic), in a RAG system admins provide the data that the system uses. This isn't as daunting as it might first sound. Often, the data you want to serve a particular use case will already exist. For example, if you want to automate third-party NDAs, you might well have a document outlining your "must-have" provisions.
One way to approach this is to consider a Venn diagram. Rather than starting only with "what kind of requests do I want to automate?" you might also consider "what kind of content do I already have?". The overlap between these two questions can define your first use case.
In other words, don't kick off a use case that responds to queries about your work from home policy if you don't already have a documented work from home policy!
Source curation is an iterative process
Once you've done an initial clean-up of the sources, it's crucial to test the outputs of your RAG system and then iterate on the source data when issues arise. A guiding principle of successful AI adoption is to learn by doing and source curation is no different.
- Ask questions that are central to your use case. This should be questions you would expect to get answered by the system, but that come in a realistic format so exactly as you receive them normally.
- Evaluate the output. The question to ask yourself here is: Am I satisfied (or even impressed!) with this answer?
- Rework the data. Most of the time, making a change to the source material can improve an answer you are dissatisfied with. The change you make will of course depend entirely on the issue with the answer, but changes often include: less ambiguous wording, a clearer delineation of the bounds of an answer (i.e. this answer is for our American entity, but not for our British one). It's worth being aware, however, that reworking the source material isn't always the answer as there be other issues at work, such as bugs.
- Repeat.
Why this is exciting
You might be thinking this all sounds like a lot of work... You wouldn't necessarily be wrong, but going back to the analogy of AI as a junior team member, you can think of this as an onboarding process of sorts. You're bringing your junior up-to-speed with the expected level of service to internal stakehodlers and how the business operates more generally.Then, once you've curated your data and the RAG system is answering well, you can sit back and relax and it responds at all hours of the day and night. It won't have a response to everything but you can step in these rare cases. Some early adopters report their RAG system as being able to handle up to 90% of incoming requests! You will certainly still encounter issues with your data - it might become redundant or out of date. But since the time taken to curate and clean it will pay off here as any issues will be easier to remedy
Content curation takes you from RAG to riches
The success of a RAG system is deeply intertwined with the quality and curation of the data it's fed. Treating your AI as a junior team member, nurturing it with carefully selected, clean, and relevant data, will not only enhance the system's accuracy and reliability but also enable it to handle an astounding number of requests autonomously. By embracing the ethos of meticulous content curation, businesses can unlock the full potential of retrieval augmented generation tools, transforming them from confused chatbots into indispensable assets that operate seamlessly at the heart of your operations.