Navigating Automation in Enterprise: Challenges, Opportunities, and Best Practices
Discover how enterprises can effectively implement automation and AI by retrofitting capabilities into existing workflows for seamless integration and high adoption rates.
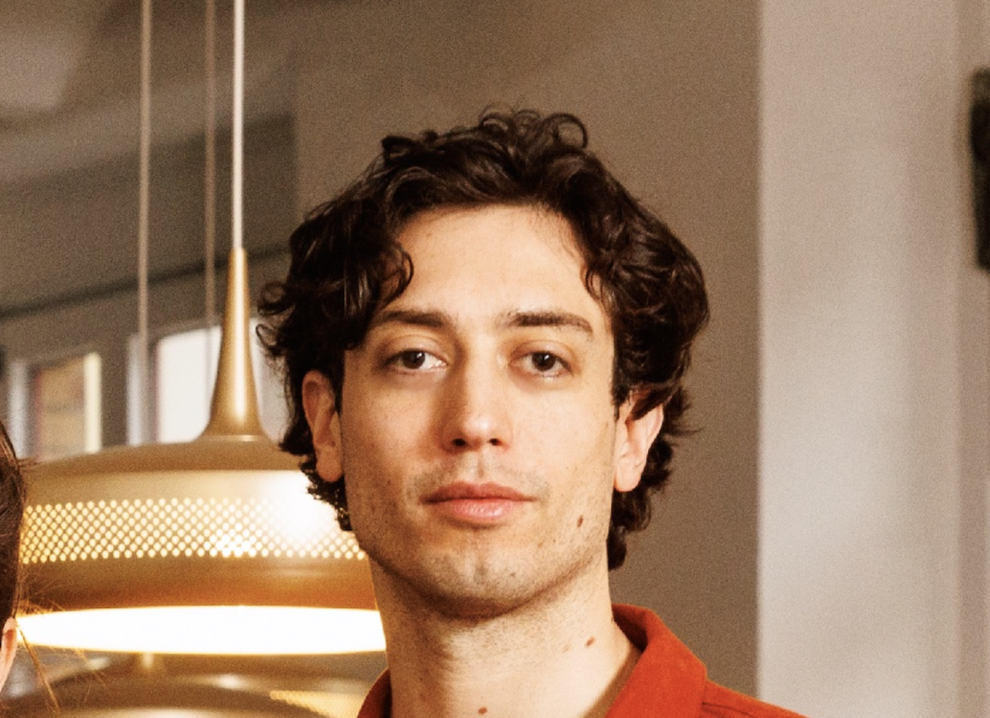
Automation and AI are transforming the business landscape, but successfully implementing these technologies in large enterprises requires a nuanced approach. Jake Jones, our Chief Product Officer and Co-Founder at Flank, shares his insights on the unique challenges and opportunities in this space. Drawing from his extensive experience across startups, scale-ups, and large enterprises, Jake provides practical strategies for integrating automation into complex organizational ecosystems.
With enterprise AI deployments, you're unlikely to have much success just “deploying a chatbot." Communication channels here are too complex (sometimes even chaotic), teams too siloed. Deploying a single central AI solution into the fragmented, unmapped terrain of an enterprise is a pipedream. There is still plenty of opportunity for automation with AI, especially for autonomous agents that can literally own entire processes and interface directly with business users. But this automation is more likely to be decentralised and embedded, an accumulation of small wins.
Let’s break this down.
The larger an organization gets, the less likely it is that there are centralized, coherent processes that span functions... You can't just plop a chatbot in there and hope for the best.
Enterprise Complexity and Siloing
The larger an organization gets, the less likely it is that there are centralized, coherent processes that span functions. More often, with enterprises, we see significant siloing of functions. Communication happens via email, direct messages, or even—god forbid—a ticketing system, and these communication channels tend to be fragmented. You can't just plop a chatbot in there and hope for the best.
It's also a lot more likely that there is software built (or at least heavily configured) by the enterprise's own IT department. So there is a (healthy) skepticism about procuring new software, especially software that cuts horizontally through the organization. "Not invented here" syndrome is a very real problem at the enterprise level. If there's been any kind of internal AI initiative in the organization already (which is typical for an enterprise), any new AI initiative (internal OR external) can raise alarm bells. To those stakeholders who led the first AI initiatives, it can appear to be a direct challenge to the work they've done.
The Complexity of Communication
Further, there are many more processes in an enterprise than in a scale-up. When you go from 2,000 to 20,000 colleagues across dozens of locations, the complexity of communication becomes difficult to follow. There are countless communication channels and processes that aren't well documented, or aren't documented at all, and are only known by those people who are directly using them.
Retrofitting: A Practical Approach
To sum this all up in a sentence: Enterprises have a mature, fragmented, and complex tech stack, so fitting new software into this context requires retrofitting rather than steamrolling. You cannot just find an existing process and say, "Let's buy a product to automate this," then get a supplier to configure it, do a bit of training, and away you go. I mean, you can try this, but what we've seen is business users won't touch it...
Enterprises have a mature, fragmented, and complex tech stack, so fitting new software into this context requires retrofitting rather than steamrolling.
A retrofitting approach works well. Rather than finding a bulky "all in one" platform, deploying it, and saying, "Here's where you go for AI!", you deploy capabilities into the tools and processes your business users are already using. In other words, there won't be one central bot people go to. There probably won't even be one single bot per function. Most likely, you'll embed a capability into an existing platform like email or SharePoint.
Use Case: NDA Workflow Automation
An example of a use case we're seeing a lot right now at the enterprise scale is the NDA workflow. This can be broken down into a few capabilities, each of which can be entirely automated with AI. There's drafting, review, and redrafting following objections. If you approach this workflow from the perspective of capabilities, rather than platforms, you can begin to automate it one step at a time.
For example, we have a couple of enterprises right now that will automate the drafting of their NDA by deploying this capability directly into email. In short, a business user emails the legal team asking for an NDA, as they have done for the past decade, and instead of the legal team gathering the information, filling out the NDA, and responding, the AI does this autonomously. It's a single capability, a single step towards automating the entire end-to-end workflow, but this capability is so seamlessly embedded into the existing system and mindset of the enterprise that adoption ends up being close to 100%.
Contrasts with Scale-ups
In a scale-up, this gentle approach is less important. There's less hesitancy towards new technology so long as it makes people's lives easier. There's also more breathing room in the tech stack. There aren't already 100 tools competing against each other. So there's less sensitivity required when positioning the AI.
Identifying Automation Opportunities
So, how do you identify opportunities for automation? Typically, we start gathering use cases by posing three questions:
1. What is the information or deliverables these business users want the most?
2. What is the information or deliverables they wait the longest to get?
3. How are they currently getting this?
Answering these questions helps identify where AI and automation can have the most significant impact, ensuring that the solutions we deploy are not just innovative but also practical and highly adoptable within the existing enterprise ecosystem.
Later, we look to identify the smallest possible implementation of the chosen use case. For example, rather than automating the entire NDA workflow for all NDAs, we first look to automate only the drafting of NDAs and only for one team. This small win is then a platform to build from, an AI beachhead.
...successful AI deployments identify the organization’s primary bottlenecks, no matter how small, within the broader complex system.
Successfully implementing automation and AI in enterprises isn’t about deploying the latest product from the biggest vendor. Instead, successful AI deployments identify the organization’s primary bottlenecks, no matter how small, within the broader complex system. Once identified, these bottlenecks should be treated in a sensitive, targeted manner, minimising disruption, and embedding deeply into the tools and processes business users are already familiar with.
Interested to find out how Flank AI agents can help your organisation? Book in a discovery call here.