How to Deploy AI for In-House Legal
Find out how to unlock the power of AI in your organisation with these practical tips on how to deploy GenAI for legal and other expert teams.
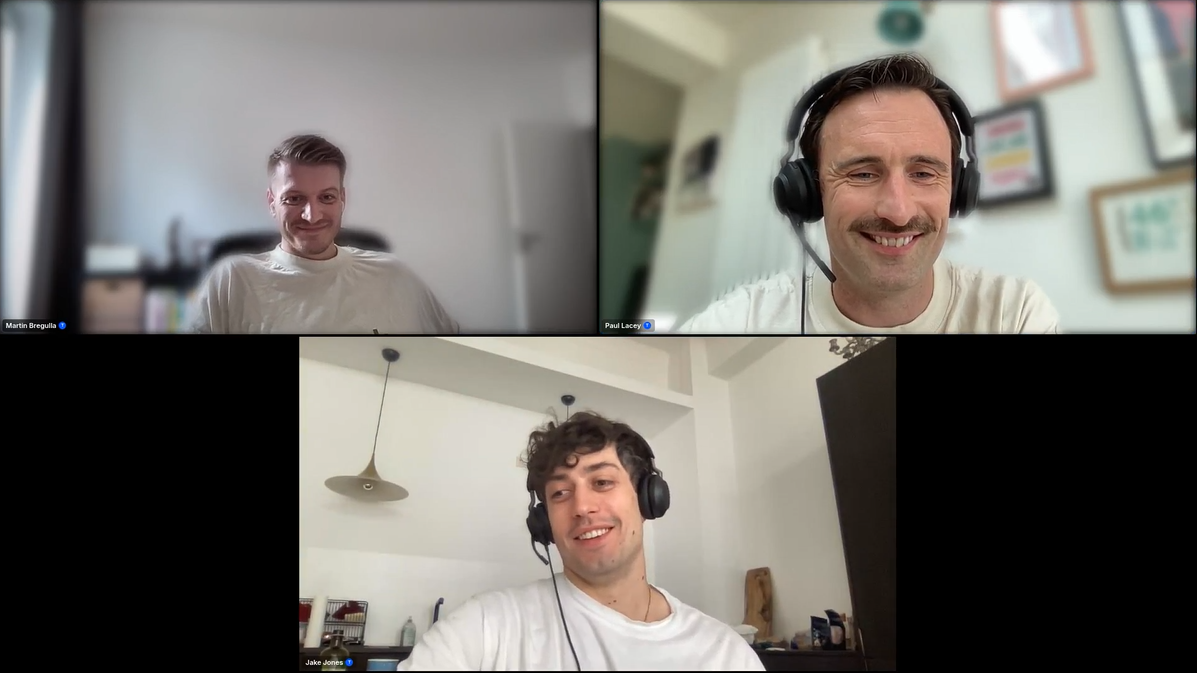
A Flank conversation on how to embed GenAI into your tech stack with Martin Bregulla, Paul Lacey, and a cameo by Jake Jones.
The opportunity we see with Flank is enabling expert teams to automate high-volume, lower-value stuff, so they can focus on the core work that requires years of training and genuine intelligence.
Martin Bregulla: Hey everyone, welcome to the webinar on general use cases for expert functions. My name is Martin Bregulla, I’m a product manager at Flank, and I’ll be hosting this webinar. Let’s kick off and bring our star Paul Lacey to the stage!
Paul Lacey: Hi Martin. Hi everyone.
MB: Paul Lacey is our Head of Product. When it comes to rolling out AI in organizations, he’s seen it all: the ugly truth, the unexpected challenges, the breakthroughs, the successes and the wins. We’ll be asking Paul all the questions about general implementation, such as Where do you begin? How do you identify a use case? How do you make sure it doesn’t go all pear-shaped? And how do you win over your team and the business as a whole? Paul, why don’t you introduce yourself?
PL: Hi everyone. I’m Paul Lacey, Head of Product at Flank. Flank plugs into company knowledge bases and deploys AI agents into the places where queries from commercial teams come to expert teams, like Slack, email, or Teams. We decided early to do both product and customer implementation together, which works really well, because we learn a lot during that process, learnings that can be reapplied to the product.
What’s changed recently is the current wave of GenAI. For the first time in a long time, it’s made people wonder if there’s now a way to solve these problems that were once considered unsolvable.
MB: Great, let’s start at the beginning. What usually triggers people to take action? What gets them to the point of thinking, we can’t carry on like this, there has to be a better way?
PL: I think we become accustomed, especially in expert teams like legal and compliance, to dealing with a very high rate of BAU (business as usual), and thinking there is no other way. We just tolerate it, and “work harder” has always been the solution to that. What’s changed recently is the wave of GenAI. For the first time in a long time, it’s made people wonder if there’s now a way to solve these problems that were once considered unsolvable. We’re seeing this a lot, and it’s being driven from various parts of the organization.
A CEO often has the headspace to look at the entire market and see bigger opportunities. Sometimes we see an AI drive from the top, because they see it as a way of creating a competitive advantage.
In some cases, it’s driven by the expert teams themselves. They feel the pain and want to resolve it, they want to serve the business better, especially if they’re getting a lot of requests from commercial teams and there’s a delay in getting back to them. But we also see it coming from operational leads that want to drive efficiency, sometimes even from the very top. A CEO often has the headspace to look at the entire market and see bigger opportunities. Sometimes we see an AI drive from the top, because they see it as a way of creating a competitive advantage. If you imagine a growth-oriented, sales-driven organization, deals are more complex than ever before, and lots of information from expert teams is needed in order to close them. The delay in getting those answers is costly, so there’s a genuine strategic advantage in using AI at the right point.
MB: Absolutely. What you say about getting lost in the BAU and working through the pain is very relatable. Especially as lawyers have a very high pain tolerance. Often they just work harder and harder, instead of improving the way they work. This is now definitely changing. I guess we’re feeling the pain more. Can you give us some concrete examples of use cases?
PL: We see lots of different use cases at Flank, from pretty basic ones that are easy to wrap your head around, to very complex ones. We work with legal and other expert teams, like compliance, finance, customer support, and technical support. Starting with legal, one we frequently see is a legal FAQ for commercial teams. Questions you might get there are Where can I find an MSA? Can you explain clause 8 of our terms to a customer? What indemnities do we offer? These are questions where there is an answer, but people don’t know where to look for it, so they just ask legal.
Linked to that we get a lot of infosec and privacy use cases. Where can I find a SOC 2 report? Can you fill in this security form for me? Likewise with RFPs. We see a lot of use cases where sales or deal desk teams are asked to fill in these enormous, incredibly time-consuming forms. In some large organizations, there’s entire teams dedicated to this. Or entity management. Where is the company’s registered address? Internal policies like HR policy. What’s the hiring process for maternity cover? Where can I find my payslip? Stuff that’s documented somewhere, but that people aren’t reading.
Then, slightly moving up the complexity scale, things like NDA review. A prospect just sent me an NDA and asked if they could sign it. Or objection handling support. A customer might object to a certain clause. The typical question here would be - A customer doesn’t like clause 4.2, our renewal clause. What can we do about it? And there’s a playbook to help you respond.
MB: That’s a really good list. What they all have in common is that it seems like work that expert functions don’t really enjoy doing. It’s not what they went through professional training for, so you’re not taking away anyone’s favorite work. So. we’re feeling the pain, we have example use cases, but how do you know that AI is the correct solution to the problems we’re facing here?
PL: The key markers we see are high-volume, repetitive requests, the kind that don’t feel like the expert’s core job. They feel urgent and important to the business, because they’re trying to close deals, but they might feel low value to the person doing them. Questions like What’s our VAT-number? It doesn’t feel like you’re making the most of your education, but if you don’t get that number, the deal’s not closing.
Another good marker is that the information is usually documented somewhere, either on a wiki, on internal websites, in documents, or in Slack channels. Slack, Teams, and other kinds of internal chats contain an enormous amount of company knowledge.
On the other hand, a use case that wouldn’t be any good for AI is interpreting the law or legislation. Maybe there’s a tool out there I’m unaware of, but that seems like proper lawyer work to me. The opportunity we see with Flank is enabling expert teams to automate high-volume, lower-value stuff, so they can focus on the core work that requires years of training and genuine intelligence.
MB: I guess a good rule of thumb would be that it’s either accessibly documented, or easily documentable. Even if it’s not yet documented, it should readily lend itself to being documented in a way that is structured and accessible to non-expert functions, which is not the case with deep legal interpretations.
PL: That’s a really good point. Documentable does not mean documented. One of the opportunities with GenAI is being able to leverage very messy, unstructured data. A principle that we hold our product to is being able to take noise and turn it into data. There’s this old-fashioned saying, according to which rubbish in means rubbish out. We intend to kill that.
Documentable does not mean documented. One of the opportunities with GenAI is being able to leverage very messy, unstructured data. A principle that we hold our product to is being able to take noise and turn it into data.
MB: Yes, we’re working hard on it. There’s probably lots of people in the audience right now that have use cases in mind, but are unsure of where to start. Is there a pecking order for which type of problem to solve first?
PL: It’s so exciting when we speak to new customers with long lists of potential use cases. That energy is really good. I always recommend to start simple. What will help more than anything is being able to demonstrate success really early on. Get buy-in and goodwill from the business. Start seeing how people adopt it, how their behavior adapts, and learn from that. Then you’re in a really strong position from which to branch out to more complicated use cases.
We suggest deploying one simple but impactful first use case. Focus on that. Keep your ‘work in progress’ low. A good example might be something like infosec and privacy, because these are usually quite well documented, out of necessity. Especially B2B businesses get a lot of repeat requests in these fields. They’re also essential for closing deals.
We’ve worked with customers on infosec and privacy use cases countless times, and we’ve seen them be deployed in days and immediately have an impact. If you start simple, you can get early traction. And once you get that initial buy-in, we then see rapid use case expansion. It kind of snowballs. The next use case is easy to deploy, because you have a willing organization. They’re not just happy to accept this new technology, they begin actively asking for more use cases.
MB: That really resonates. People can be a bit hesitant about those early, simple use cases, because they feel like they’re too low value. They’re buying a fancy AI product and immediately want the very complex stuff, but these simple use cases are actually very high value for the commercial teams. And then you get a snowball effect, which allows you to work on more complex use cases afterwards.
So, once you’ve selected your first use case and got it rolling a bit, what is the secret sauce? What key ingredients do you need for a successful use case?
People can be a bit hesitant about those early, simple use cases, because they feel like they’re too low value. They’re buying a fancy AI product and immediately want the very complex stuff, but these simple use cases are actually very high value for the commercial teams. And then you get a snowball effect, which allows you to work on more complex use cases afterwards.
PL: The teams that do best are the ones that, working very closely with us, work back from the intended audience and their problem. It’s not always helpful to frame a use case from your own expert perspective. Expert teams often categorize their work into different areas of expertise, and your end user may not see the world that way. Really getting into your end user’s mind and understanding their approach is a good way to ensure success. Have a target persona in mind. An example of a target persona would be a customer success agent trying to answer product questions coming in from customers. That information allows you to better bound the use case, understand what content can drive it, what kind of queries you’re going to get, who you need to roll it out to, and where they’re already asking these questions.
The second point that links from that one is to involve your target persona as soon as possible. Don’t try to roll it out with a big bang. Create a beta group who can start asking it questions. Don’t spend too much time testing it internally. Our Flank agents work pretty much straight out of the box. Of course people want to check things, that’s only natural, but do it with real users. We find that experts ask questions in a slightly different way, because they already know the answer. They inadvertently or deliberately try to either draw out the answer and help the agent, or trip it up and really test it. Testing with real users works best. Any issues or gaps in knowledge surface really quickly that way. And you get enthusiastic users too, who help you roll out and champion the product.
I really recommend treating it like a product rollout. Tell people why you’re doing this. Give them information. We have tons of content that we share with customers to help them through the early days. A core product principle is how it lands in the early days is a massive indicator of later success. When you first roll it out, focus your time on being around to support, answer any unknowns, and really advocate for it. You’ll find that everything else becomes easy. We’ve seen teams that, after focusing a lot of effort at the beginning, are now almost completely hands off.
The teams that do best are the ones that, working very closely with us, work back from the intended audience and their problem. It’s not always helpful to frame a use case from your own expert perspective.
MB: That’s a really good perspective. Treating it as a product rollout also doesn’t mean you need a product background, just a product mindset – being very close to your internal customer and wanting to do the best for them. If you work in an expert function, that’s what you’re trying to do already. What we see is that, if teams treat this as a product rollout, user satisfaction goes up.
PL: The point on user satisfaction is actually really interesting and kind of counterintuitive. One of the concerns we hear from expert teams is that automating these high-frequency requests will disconnect them from the business, that they’ll go back to working in their little expert silos. But in fact, it’s quite the opposite. Some of our customers have even done surveys on this. They show that automating these requests frees up time for the expert teams, which can be invested in building relationships with people in other departments.
Commercial teams are now getting their high-frequency requests answered really quickly. For the more complicated requests, where the experts can really use their heavyweight expertise, they’re now on hand to do that much quicker. So satisfaction goes up across the board, but expert and commercial teams also feel closer, more connected to each other. It’s a massive opportunity.
One of the concerns we hear from expert teams is that automating these high-frequency requests will disconnect them from the business, that they’ll go back to working in their little expert silos. But in fact, it’s quite the opposite. Some of our customers have even done surveys on this. They show that automating these requests frees up time for the expert teams, which can be invested in building relationships with people in other departments.
MB: And not just that. It feels like people were afraid to bother their expert functions with these questions before. Now that they know it’s not a human answering, they ask more questions, but they probably had these questions before.
PL: There’s a slightly scary side to that as well. We’ve seen data where automation doubles the number of queries being asked. Who was answering them before? No one was. That’s a hidden cost of not doing this already.
MB: And that hidden cost just increases with the complexity of the organization. Considering which use cases are impactful and how they’re implemented, have you noticed any differences between large enterprise companies and smaller scaleups?
PL: I thought you might ask this, so I’m going to invite Jake Jones up to answer for me. Jake is one of the co-founders of Flank, and probably best placed to answer this question.
Jake Jones: Anyone who’s ever deployed software to enterprise companies knows it’s a totally different world. In the simplest terms, at an enterprise you won’t get away with just deploying a chatbot. You won’t have any success like that.
Firstly, you won’t have any central place you can deploy it to. Enterprises are a very different technological landscape. They have very different tech stacks and very different challenges. The larger an organization gets, the less likely it is to have centralized, coherent processes that span across functions. More often, with enterprises, we see a lot of siloing of functions. For example, there’s rarely a central MS Teams or Slack instance that all teams collaborate on. If there is a central instance, it’s not being used in the open, collaborative way we see at scaleups, where it’s almost a public discussion. Instead, the conversations tend to be through email or direct messages, both very siloed and fragmented communication channels. So there’s no obvious place to plug in a chatbot. Not that this is necessarily the mindset you should adopt at a scaleup, but certainly not at an enterprise.
Secondly, at an enterprise it’s a lot more likely that there were previous AI initiatives. So there’s often healthy skepticism about any new initiatives, especially from those people responsible for launching the initial ones. These people are very important. They’ve laid the foundation, made the first steps. They’re pioneers. But they can also be nervous about people reinventing the wheel, coming in and wanting to do AI differently. To them, it can feel like that’s what’s happening when new AI initiatives are being launched.
But it’s not all doom and gloom. There are approaches we see working really well. Currently, I’m just laying out the challenges of scale. When you go from 2,000 to 20,000 colleagues, especially in a global organization with dozens of locations, the complexity of communication does proliferate. To sum it up in a sentence, you get a much more complex, mature, and fragmented tech stack that you’re trying to embed AI into. So, when you’re looking for use cases, you can’t just find an existing process, buy a product for it, and get a supplier to figure it out. Instead, you need to take an approach of retrofitting.
You need to deeply understand the business users, how they work, what their highest priorities are, what they want, and what’s slowing them down. Then, rather than finding a bulky, all-in-one platform for a whole expert function like legal, compliance, or security, you can look at those specific needs and ask where an AI capability could be plugged in. Even if it’s just one really narrow thing, it would mean never having to think about it again. It will plug into the process forever.
A good example, and we see this all the time, is an email process for NDAs or basic supplier terms. How many times a month do people in an enterprise request an NDA? I know for a fact, in some of the enterprises we’re working with, it’s in the many hundreds. I can imagine, for a lot of enterprises it’s in the thousands, not to mention the review and redrafting process, the back and forth. What would happen if, rather than having to wait, you got your NDA immediately? The bot would find the right NDA, populate all the fields, and get all the right information from the business user. These are the kinds of opportunities you want to look for when finding your first use case for an enterprise. Take a single capability, like drafting an NDA, plug it into a tool, in a place that’s already being used, and deploy it in such a way that it’s almost invisible to the organization.
At enterprise level, it becomes much more of a process mining activity, less of an enjoyable procurement activity where you buy some new, sexy SaaS tool and plug it in. That’s not going to happen. If you try that, my bet is - it will fail. These are our experiences so far. In a year we could see this changing, but for now this is pretty accurate.
If you found this useful or have lots of questions, follow us on LinkedIn or find more information about us on our website, where you can also book a demo. Just reach out and we’ll help you find a good use case to start your own GenAI journey with.
You can read about Lusha's, PROS', TravelPerk's or QA's journey with AI on our blog.